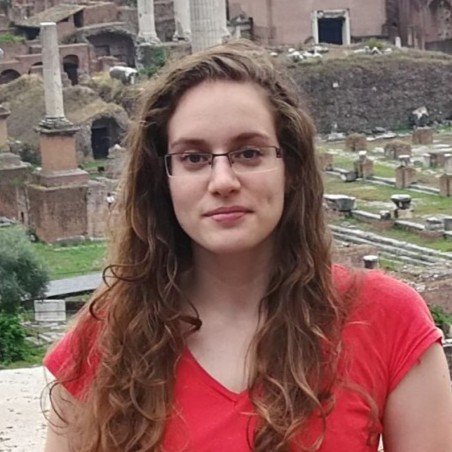
Hila Manor
I am a PhD candidate at the Electrical and Computer Engineering department of the Technion, under the supervission of Prof. Tomer Michaeli.
I received my BSc (Summa Cum Laude) in Electrical and Computer Engineering at the Technion as well.
My research interests lie in the field of deep learning, with a focus on generative models. I am particularly interested in exploring their applications, interpretability, and reliability.
You can contant me at hila dot manor at campus dot technion dot ac dot il
.
Publications
Hila Manor, Tomer Michaeli
We presents two methods for zero-shot editing of audio signals using pre-trained diffusion models, for both general audio and music signals. The first method uses text guidance to modify the signal according to a desired text prompt. The second method involves discovering semantically meaningful editing directions in the noise space of the diffusion model in an unsupervised manner, based on our previous work.

Hila Manor, Tomer Michaeli
We presents a novel method for estimating and visualizing the uncertainty in image denoising tasks, using only a pre-trained denoiser and its derivatives. We first derive a relation between the higher-order posterior moments and the higher-order derivatives of the posterior mean, which is the output of the denoiser. Then we use this relation to efficiently compute the principal components of the posterior covariance, as well as to approximate the full marginal posterior distribution along any 1D direction. Our method reveals meaningful semantic variations and likelihoods of the possible reconstructions, in a variety of domains and denoiser architectures.


Noa Cohen, Hila Manor, Yuval Bahat, Tomer Michaeli
We observe that current image restoration algorithms do not fully capture the diversity of plausible solutions. Following that, we initiate the study of meaningful diversity in image restoration. We explore several post-processing approaches for uncovering semantic diversity in image restoration solutions. In addition, we propose a method for diversity-guided image generation using diffusion models.

Teaching
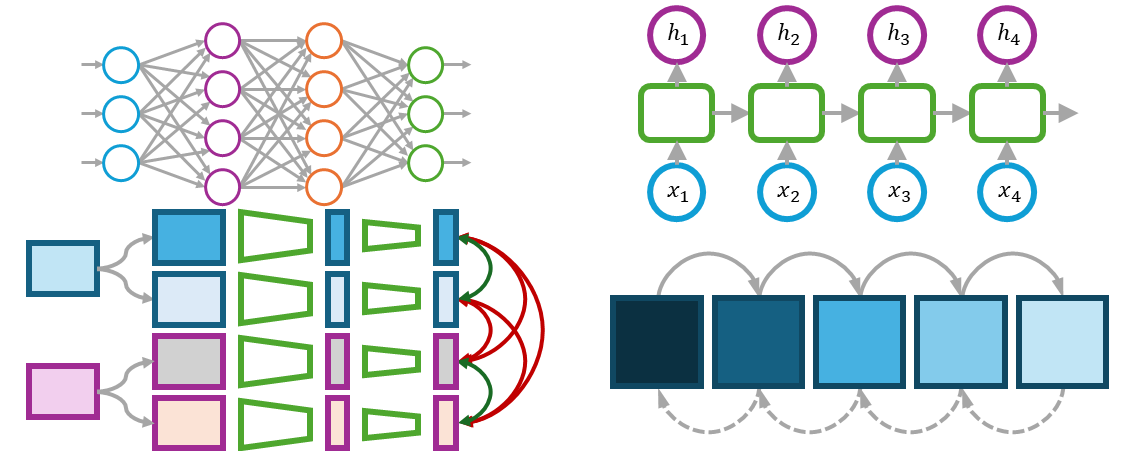
Deep Learning Lab
Spring 2023, Winter 2023-24
The lab aims to develop a comprehensive understanding of various fields in deep learning through hands-on experimentation. We explore the basics, CNNs, self-supervised learning, NLP, and diffusion models.
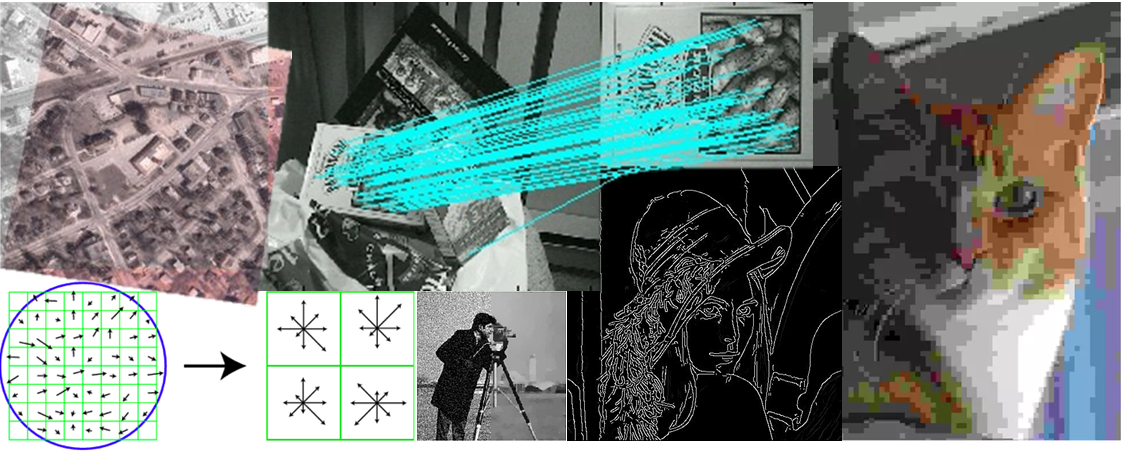
Image Processing Lab
Winter 2023-24
The lab is focused on classical image processing, and provides practical hands-on experience covering a range of concecpts, such as compression, image registration, and total variation techniques.
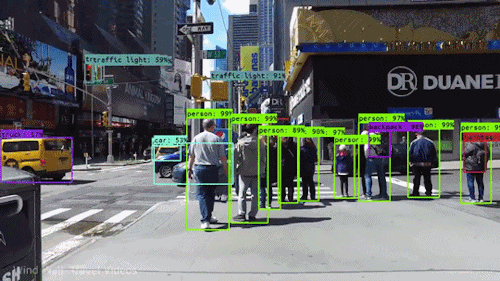
Winter 2022-23, Spring 2023, Winter 2023-24
Head TA. Taught in English alongside Elias Nehme, Moshe Kimhi, Prof. Anat Levin, and Prof. Lihi Zelnik-Manor.
The course focuses on fundamental problems in computer vision describing practical solutions including state-of-the-art deep learning approaches.
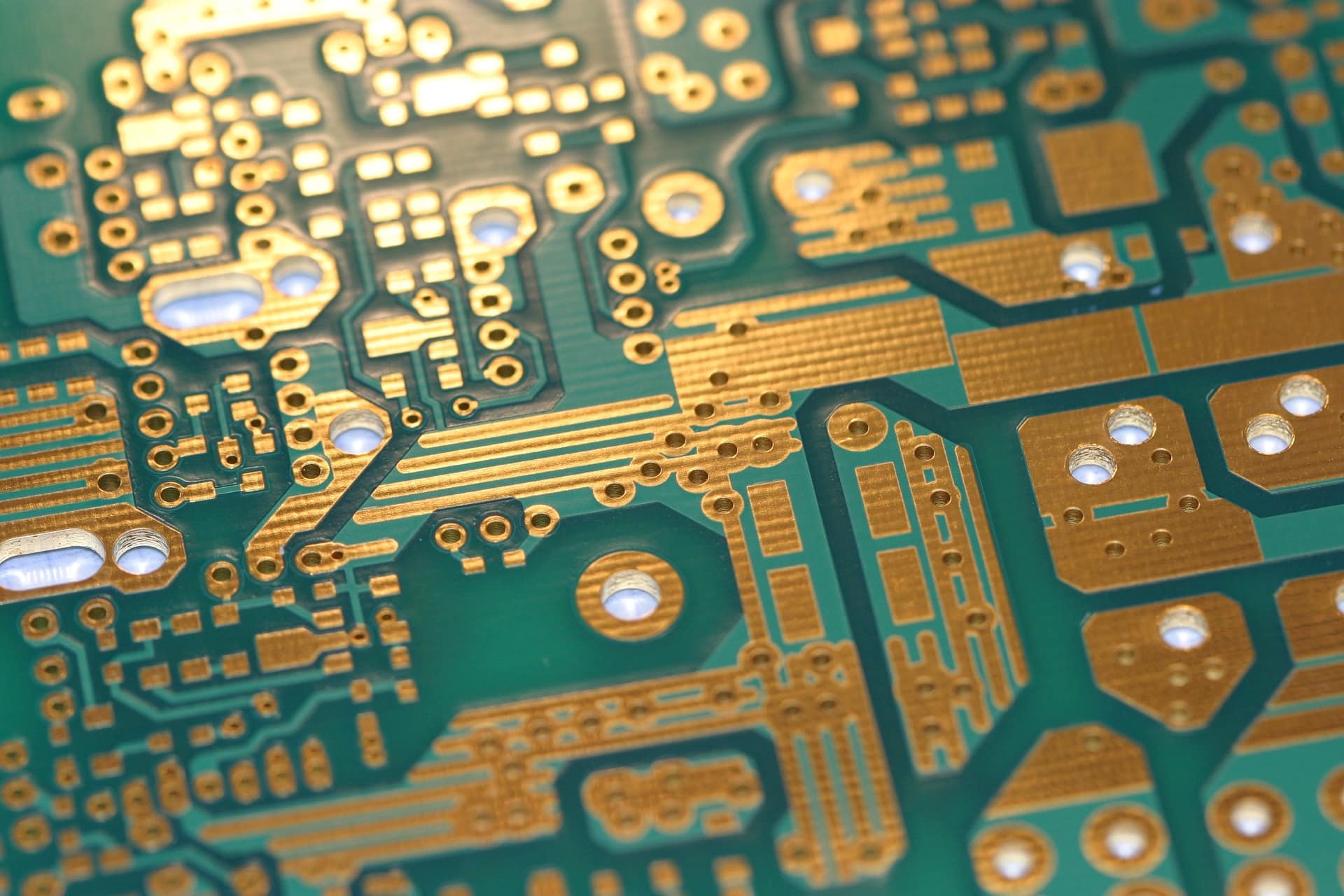
High-Speed DSL Lab
Winter 2022-23, Spring 2023, Winter 2023-24
In the lab we explore signal integrity on transmittion lines in PCBs, by investigating reflections of signals and jitter, both in simulation and on physical boards.